Introduction to Methodology
The methodology involves the study of research methods that includes a contextual framework based on a coherent and logical scheme based on views. This section creates a primal road map on how to conduct the research and get the nest answers to the research section. This study will adopt both qualitative and quantitative research methods. The data collected will then be analyzed and presented in a manner that can be understood by the audience. Data analysis’ aim is to give meaning to the data collected. Furthermore, this section discusses the research design that involves the overall strategy chosen to integrate the various study components coherently and logically. Consequently, the research design effectively addresses the research problem since it consists of the data collection blueprint, measurement, and analysis (Chowdhury and Shil, 2021).
The data collection method in this study involves gathering and measuring information on the study’s targeted variables. Therefore, the data collection phase allows the researchers to answer the study’s relevant questions and evaluate the outcome (Newman et al., 2021). This study will involve various data collection techniques with an emphasis on an online survey, document reviews, interviews, and focus groups. The survey will carry out on selected SMEs and big corporations in China. The data collection method presents various advantages and disadvantages that are discussed in-depth. The face-to-face interview involves the tracking of consumer-company interactions as enhanced through the use of AI in business activities by asking the stakeholders questions physically. Meanwhile, online interviews and focus groups will involve asking set questions to selected stakeholders within the business organizations under study.
Data collection is a social process that will involve interacting with various people holding different cultures. Therefore, the researchers will use sampling techniques to sieve out credible respondents. Furthermore, the recruitment process will be purely ethical, and in tandem with China’s and international data and information laws. The researchers are guided by a chosen code of ethics during the data collection, analysis, and recording. This study considers the data integrity issues to support errors’ observations during data collection. The study applies the two approaches as invented by Craddick, Crawford, Rhodes, Radican, Rukenbrod, and Laws in 2003 (Kask, Piho, and Ross, 2021). Therefore, data quality assurance and control are carried out in this study.
Qualitative Data
The study’s qualitative data provide a descriptive and conceptual finding collected through questionnaires, online interviews, face-to-face interviews, and document reviews. The data include textual responses collected from the companies under study and from the various social media platforms. Therefore, this study’s qualitative data includes the narratives, stories, and experiences of the chosen study sample. Qualitative data analysis will allow the researchers to explore ideas and explain numerical data collected (Maher et al., 2018). The qualitative approach presents various advantages and disadvantages.
Advantages of Qualitative Approach
The approach can help the researchers collect and analyze changing attitudes within the sample group including the employees’ attitudes towards AI integration.
The qualitative data help the researchers have an explanation of something number alone cannot reveal. For instance, the impact of AI on SMEs needs a sharing on consumer experiences and companies’ brands’ image observation
The qualitative data will allow the researchers to promote data capture by their ‘gut feel’ for where to collect good information
Disadvantages of Qualitative approach
Since this research involves a large sample population, the qualitative data collected may not provide a true reflection of the views of the many companies and SMEs that have adopted AI integration
The research qualitative data is subject to bias due to competing agendas among the various SMEs and large corporations in China. The chosen sample respondents may be consciously and unconsciously influenced to answer questions and give responses that favor their companies.
Posing wrong questions may lead the researchers to miss crucial information.
Quantitative Data
Although this study utilized a qualitative approach, quantitative data were used to supplement the qualitative data collected. The quantitative data used in this research included the financial reports of the selected companies in China. The financial data helped the researchers track the profitability of AI integration among the business activities in China. Furthermore, the quantitative collected included the number of years the companies adopted AI integration. Consequently, the researchers were able to determine the duration of AI usage among companies and SMEs in China. The quantitative data collected would come in handy for the companies intending to adopt AI in their activities since they can estimate the profitability of the AI. The quantitative data collected have various advantages and disadvantages (Djafar et al. 2021).
Advantages of Quantitative Data
The quantitative data collected uphold the anonymity of companies and SMEs taking part in this research. Consequently, the research participants gained the confidence needed to reveal vital information.
The given data is trustworthy and repeatable, unlike the qualitative data that is subject to the personal views of the employees in the sampled companies.
The quantitative data employ a random model to capture raw data eliminating all kinds of bias and favoritism.
The quantitative data also saves the research duration since the data collection method employed is faster than in the collection of qualitative data.
Disadvantages of Quantitative Data
The conclusions made might be irrelevant to the overall research question which is the impact of AI on business activities
Unlike the qualitative data, the quantitative data give no room for follow-up. Consequently, the data cannot be used to ask further questions that may arise in the course of the study.
Like qualitative data, the numerical data is subject to biases since the companies may want to expose their profits and hide the losses from the researchers.
The qualitative and quantitative data collected will help serve various purposes in the course of this study. The overall significance of the data is answering the primary research question, “what is the impact of AI integration among the companies in China?” Furthermore, the data collected would help in achieving the research objectives. Data analysis will help understand the research problem presented and draw a substantive conclusion that can help build upon further research. Therefore, the mixed data-type approach is significant to this study since it will help serve different purposes in the study as summarized in figure 1.
Research Design
The research design employed in this study is the qualitative case study involving companies in China. The qualitative research design would help in achieving the aim of the study which is to understand and explain the impact of AI on the various business activities and management among companies in China. The design ensures that the evidence obtained, data, allow the researchers to effectively address the research problem (Trang, 2022). Furthermore, the research design would help develop logical and unambiguous conclusions (Stewart, 2022). Upon deciding the overarching research design, a research strategy ought to be chosen to explain and described the main research questions and objectives (Thompson and Schick-Makaroff, 2021).
The research model is based on the previous research conducted on the impact of AI integration on business activities. According to Brown, Burke, and Sauciuc (2021) artificial intelligence has transformed business activities and employees. Through, AI the employees have been positively motivated since much of their work is automated to enhance efficiency. Although AI has created jobs for professionals like software development, many employees have lost their jobs (Malik, Tripathi, and Gupta, 2021). Meanwhile, AI has impacted various company activities leading to improved quality output, increased profits, and improved competition (Mikalef, Conboy, and Krogstie, 2021). While the initial research generalized the impact of AI on employees and companies, this study utilizes the collected data to establish AI impact among companies in China. Therefore, the qualitative research design helps build a logical research model guiding the researchers during the study.
Data Collection
Since this study employs a qualitative research design, qualitative data collection methods are used to gather the necessary information relevant to the study topic. Data collection is the most valuable part of research; therefore, the process should be logical and done in a manner that utilizes the existing research tools (Flick, 2018). This study conducted logical steps to ensure that the data collection process was effective. Firstly, the researchers determined the type of data intended to be collected and the sources of the data. Secondly, a timeframe for data collection was set to ensure that no single data was excluded during the process. Setting a timeframe during data collection is beneficial since it enhances the credibility of collected data and ensures that no time is wasted during the process (Gill and Baillie, 2018). Finally, the data collected was rigorously filtered to eliminate any overt bias. The data collection methods used include focus groups, online interviews, surveys, document reviews, and face-to-face interviews.
Focus Groups
The SMEs in China are mostly sole proprietorships and the owners can be approached with ease. This study involved various SMEs owners who carry out their business activities in China. Due to limited research scope and time, the researchers conducted focus groups among the small business owners in Beijing. The participants were given set questions which they were required to respond to as a group. The use of focus groups helped collect information on collective views and the meanings that lie behind those views (Nyumba et al., 2018). The study considered Morgan’s views on limiting the use of focus groups (Morgan, 2018). Therefore, the participants were free of unease and had no differences from each other. Group mix was used to avoid data biased of gender, age, and professional statuses.
Interviews and Online Surveys
Interviews are the most effective for qualitative research since they help in understanding, explaining, and exploring research subjects’ opinions, behaviors, experiences, and phenomena. Moreover, interview questions can be open-ended so that in-depth information can be collected (Ballena, 2021). There are three fundamental types of interviews: structured, semi-structured, and unstructured (Osborne and Grant-Smith, 2021). Structured interviews are verbally administered with a list of predetermined questions (Osborne and Grant-Smith, 2021). Meanwhile, unstructured interviews do not reflect preconceived theories and are performed without organization (Makri and Neely, 2021).
Therefore, this study utilizes structured interviews to collect information from the selected respondents. The structured interviews are relatively easy and quick to administer (Mogaji et al., 2021). The interviews are also simple to use if there is clarification needed and if the respondents have literacy and numerical problems (Mogaji et al., 2021). However, structured interviews allow limited participation and are of little use if an ‘in-depth’ response is required. This study uses structured behavioral interviews and structured situational interviews. The structured behavioral interview helps the researchers learn about the employees’ behaviors past experiences and how they influence their working motivation. Alternatively, structured situational interviews help ask hypothetical questions about how they would respond to certain situations in the absence of AI.
This study uses online Google forms to collect data from the respondents. The forms have predetermined questions that are close-ended and some are open-ended. The data filled in in the Google Forms are then stored in a cloud-based database that researchers can access (Melo, 2018). The main advantage of using Google Forms is that they can be set to allow a single response and the data collection timeframe can also be set. Google Forms also have a notification option that allows the researchers to have a real-time response (Melo, 2018). Furthermore, the forms have inbuilt data analysis tools including graphs and pie charts (Melo, 2018). The researchers can automatically analyze the responses and observe the trend (Melo, 2018). Consequently, Google Forms are a viable data collection tool for collecting information from the research participants.
While the Google forms are beneficial to the study, they have several disadvantages that limit the data collection process. The forms need a stable internet connection to be used; thus, the respondents without an internet connection may find it difficult to fill in the forms (Melo, 2018). The forms also need computer literacy including the creation of Google accounts to have access to the forms. Furthermore, unlike the physical forms, the Google Forms have textual and image size limitations: accepts texts up to 500 kilobytes, images up to 2 megabytes, and a limit of 256 cells or 40 sheets for spreadsheets (Melo, 2018). This study utilizes physical questionnaires, replicas of the Google forms, to collect data from the respondents without the ability to use smartphones and Google Forms. Therefore, the use of both online and physical questionnaires helped alleviate the Google Forms limitations.
Conducting Face to Face Interviews
Face-to-face interviews can be troublesome since they require the researchers to work on a fixed schedule and make an appointment before the meeting. The researchers engaged with the key decision-makers who were interviewed online and offline according to their preferences. The researchers had predetermined questions for the interviewees that gave led to the major research questions and objectives. Time limitation led the researchers to engage with fewer interviewees (Saarijärvi and Bratt, 2021). Furthermore, some intended interviewees failed to accept the interview request, limiting the amount of data collected. Although the face-to-face interviews presented time and population limitations, the data collection method was significant since the researchers could collect more information than using physical forms (Saarijärvi and Bratt, 2021). Furthermore, intercepting with the respondents allowed the researchers to observe the respondent’ behaviors and attitudes towards AI integration among companies and SMEs in China. Face-to-Face interviews were crucial to the study since they enabled collection of the information that could not be collected using Google forms.
Document Reviews
Many scholars have written on the thematic area of study, and the information is crucial for this research. Furthermore, some Chinese companies publish their information and annual reports online. Consequently, some of the reports can be downloaded and printed for review. The document review involves analysis of existing written reports and written works on the topic (Gómez, Roncancio, and Casallas, 2021). The companies’ reports would allow the researchers to collect financial reports and companies’ performance reports. Furthermore, existing research articles will enable data collection of the population not covered in this study. The main advantage of document analysis is the facilitation of a large amount of information without engaging many respondents (Subekti, 2021). However, document analysis is a secondary method of data collection and is likely to have some errors (Gómez, Roncancio, and Casallas, 2021). A mixture of document analysis, online surveys, face-to-face interviews, and focused groups allows the researchers to collect sufficient data for the study.
Data Integrity issues
Data intercity involves the overall accuracy, consistency, and completeness of the collected data. This study’s data collection is collected online and could lead to integrity issues. The data integrity issues include the filling in of inaccurate information and the unintended respondents taking part in the research. According to Jones et al.,2021 qualitative data collection occurs online and lacks a synchronous contact between the researchers and the participants as present in the face-to-face interviews. Therefore, there are challenges concerning participant eligibility and data quality in the qualitative domain (Jones et al., 2021). Therefore, this study included various techniques to ensure data quality.
Quality Assurance and Control
The study utilized the techniques, systems, and resources to give an assurance about the care and control with which the research was conducted. The researchers had a research schedule that defined the various roles of the participants and any other party involved in the research. Although the number of research staff was less than three, the staff had allocated roles that were trained. This study also utilized dependable facilities and equipment during data collection. The researchers created a specialized e-mail account for any research communication and creation of Google forms. The separate e-mail account enabled the researchers to avoid mixing personal information with that of the research. While a separate e-mail account helped avoid data mix-up, the utilization of Google forms data-entry restriction options enabled the researchers to control the type of data entered from a restricted participant.
Furthermore, the document analysis was conducted on documents and articles that were obtained from reliable sources. The sources included reputable journals’ databases and the companies’ genuine websites. The reputable journals’ databases used included CORE, ScienceOpen, SAGE, and ScienceDirect. The researchers ensured that the financial reports analyzed were obtained from the companies’ legitimate websites and were stamped by the companies’ accountants. Although ensuring data quality during the face-to-face interviews was problematic, the researchers ensured that the respondents were warmly accommodated and there were few external interruptions.
The study had an established clear data normalization protocol that enabled the researchers to have a clear sense of how data was collected and recorded. Normalizing refers to standardizing all data features and categories making the data collection process clear and easy (Griffin et al., 2021). The study had a research plan with clearly defined data collection protocols before data collection. Furthermore, the collected data had a consistent format and standardized measurements. The selected data collection and handling tool, Google Drive, is consistent and promotes data integrity including security features like the use of passwords (Atlam and Wills, 2020). The data quality assurance and control as manifested in the study presented some benefits.
Quality assurance and control during data collection are significant to the research and the intended audience. Integrity during data collection enables the production of reliable data that yields rigorous and dependable results (Rose and Johnson, 2020). The university’s code of research conduct firmly recommends quality assurance during data collection and research conduct. This study is in tandem with the set rules and guidelines on mandatory quality data assurance. Furthermore, quality data assurance and control help the researchers comply with existing Chinese and integrational laws on data and information privacy (Determann, 2019). Quality assurance was significant during the data collection since it allowed compliance with institutional, Chinese, and international laws on data integrity and privacy.
Recruitment and Sampling
The overarching thematic area includes various stakeholders and many businesses without the research scope. This study is limited to the number of participants and finance to conduct the research. The participants were subjected to a rigorous recruitment process making the research viable and cost-effective. The recruitment process is significant during research since it allows the researchers to categorize the participants and filter out those who could lead to erroneous data (Zwan et al., 2021). This study used the purposive sampling technique to categorize the participants in terms of hierarchy, diversity, business size, and location.
Hierarchy consisted of the various roles played by the employees within organizations. The roles were divided into three categories: top-management, middle-management, and front worker. The top-management participants included the departmental managers and supervisors while the middle-management involved the group leaders. Meanwhile, the front workers included all those employees without leadership roles. Categorizing the participants according to the hierarchy allowed the researchers to get an overview of the impact of AI integration on business management and general business operations. Furthermore, hierarchy categorization made the research reliable since the data collection comprised of the whole business perspective.
Diversity is crucial among the participants since China is commercially diverse. The participating companies were categorized according to their country of origin: international or local company. Companies registered under the Chinese company laws were considered local companies, while those registered in other countries and operated in China as subsidiary or multinational enterprises were considered international companies. Furthermore, the companies were categorized as manufacturing, service, or processing companies. Since technology plays a crucial role in manufacturing, processing, and service industries, categorizing companies according to diversity gave a full picture of the role of AI in business activities.
China is a big country with a large population making it difficult for the research to include all companies in the country. Consequently, the research participants were also categorized according to their location. Although many companies are located within China’s big cities such as Beijing, Weifang, Harbin among others, some companies and SMEs are located in the rural areas. Therefore, the participating companies were categorized according to their location: urban and rural. The rural-urban categorization helped the researchers identify which areas are likely to integrate AI within their business activities and management. The companies were further categorized according to the business size: SMEs and large corporations.
The study’s recruitment process was vigorous and ensured that all categories were evenly covered during data collection. The diverse companies’ categories allow the research to provide wholesome and reliable information that could lead to further research. However, the study was subject to generalization given the wide scope of the participating companies and respondents. The total number of the respondents were as summarized in Figure 3.
The research involved a total of 84 participants representing the various companies’ categories in China. Out of the 84 participants, 78 were working in registered companies in China and at international levels. Among those working in companies, the 15 top managers were subjected to either face-to-face interviews or online interviews. The remaining 63 participants were subjected to online Google forms that were sent to them via unique links. The 6 SMEs participants were allowed to either use the Google forms or fill in the printed forms with similar questions.
Ethical Concerns
The study is conducted within the Chinese jurisdiction that has strict laws on data privacy and protection. Therefore, the research is conducted strictly by the Chinese laws on data protection. The participants’ personal information will not be revealed to any party whatsoever. Protecting the respondents’ personal information helps the respondents give truthful and dependable information without fear of vindication. Anonymity among the participants including concealment of information such as company name, job position, and employee name are the primary terms on the contract of participation. The respondents were required to sign a participation contract that ensured their data protection.
Furthermore, the researchers’ behavior during and after the data collection was of great importance. The researchers were required to hold themselves to a high-level o of decorum and avoid raising issues that would violate the respondents’ personality rights. The researchers ought to avoid asking questions that attacked the family and dignity of the respondents during the face-to-face interviews. Moreover, the researchers were restricted from sharing the respondents’ responses with any party including the participating companies. Conducting ethical research is beneficial since the respondents and the audience gains trust in the study and might end up revealing crucial information that is significant to the study (Iphofen and Tolich, 2018). Therefore, this study was ethically justified and was conducted per data protection and privacy laws in China making it reliable.
Data Analysis
The collected data were subjected to analysis and interpretation to get meaning. This study utilized the Creswell data analysis model that allows data validity, accuracy, and effective interpretation (Figure 4.). The Creswell model allowed the researchers to analyze the raw data, validate the accuracy and align the results with the thematic area of research. The raw collected during the research were recorded interviews and the questionnaire responses. The data was then presented using graphs and pie charts.
Methodology Conclusion
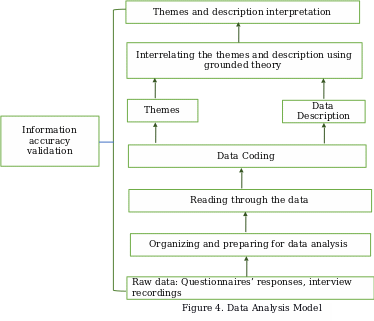
The research methodology gives a road map of the data collection process and the data analysis. The chapter outlines the various data collection methods and the intended participants. This research categorized the participants into various categories to give meaning to the data collected and improve the research scope. The study utilized a mix of data collection methods including focus groups, online interviews, and the use of questionnaires. Data integrity was ensured through data quality control and assurance. The participants and the researchers were strictly under a code of ethics to ensure every participant was accommodated. The research methodology is justified since the data collection and analysis tools were effective.
References
Atlam, H.F. and Wills, G.B. (2020) IoT privacy security safety and ethics. In Digital twin technologies and smart cities (pp. 123-149). Springer, Cham. Web.
Ballena, C.T. (2021) ‘Qualitative Research Interviewing: Typology of Graduate Students’ Interview Questions’, Philippine Social Science Journal, 4(3), pp.96-112.
Brown, J., Burke, J. and Sauciuc, A. (2021) ‘Workforce Diversity and Artificial Intelligence: Implications for AI Integration into Performance Evaluation Systems’, SSRN, Web.
Chowdhury, A., & Shil, N. C. (2021) ‘Thinking Qualitative Through a Case Study: Homework for a Researcher’, American Journal of Qualitative Research, 5(2), 190-210.
Determann, L. (2019) ‘Healthy data protection’ Mich. Tech. L. Rev., 26, p.229, Web.
Djafar, H., Yunus, R., Pomalato, S.W.D. and Rasid, R. (2021) ‘Qualitative and Quantitative Paradigm Constellation In Educational Research Methodology’, International Journal of Educational Research & Social Sciences, 2(2), pp.339-345. Web.
Flick, U. (2018) ‘Triangulation in data collection’, The SAGE handbook of qualitative data collection, pp.527-544. Web.
Gill, P. and Baillie, J. (2018) ‘Interviews and focus groups in qualitative research: an update for the digital age’, British dental journal, 225(7), pp.668-672. Web.
Gómez, P., Roncancio, C. and Casallas, R. (2021) ‘Analysis and evaluation of document-oriented structures’, Data & Knowledge Engineering, 134, p.101893. Web.
Griffin, M. et al. (2021) ‘Ensuring survey research data integrity in the era of internet bots’, Quality & quantity, pp.1-12. Web.
Iphofen, R. and Tolich, M. eds. (2018) The SAGE handbook of qualitative research ethics. Sage.
Jones, K. (2021) ‘Challenging issues of integrity and identity of participants in non-synchronous online qualitative methods’, Methods in Psychology 5.
Kask, M., Piho, G. and Ross, P. (2021) ‘Systematic Literature Review of Methods for Maintaining Data Integrity’, International Conference on Model and Data Engineering (pp. 259-268). Springer, Cham.
Maher, C., Hadfield, M., Hutchings, M. and De Eyto, A. (2018) ‘Ensuring rigor in qualitative data analysis: A design research approach to coding combining NVivo with traditional material methods’, International journal of qualitative methods, 17(1), p.1609406918786362.
Malik, N., Tripathi, S.N., Kar, A.K. and Gupta, S. (2021) ‘Impact of artificial intelligence on employees working in industry 4.0 led organizations’, International Journal of Manpower.
Melo, S. (2018) Advantages and Disadvantages of Google Forms. [online] DataScope. Web.
Mikalef, P., Conboy, K. and Krogstie, J. (2021) ‘Artificial intelligence as an enabler of B2B marketing: A dynamic capabilities micro-foundations approach’, Industrial Marketing Management, 98, pp.80-92. Web.
Mogaji, E., Balakrishnan, J., Nwoba, A.C. and Nguyen, N.P. (2021) ‘Emerging-market consumers’ interactions with banking chatbots’, Telematics and Informatics, 65, p.101711. Web.
Morgan, D.L. (2018) Basic and advanced focus groups. Sage Publications.
Newman, A., Bavik, Y.L., Mount, M. and Shao, B. (2021) ‘Data collection via online platforms: Challenges and recommendations for future research’, Applied Psychology, 70(3), pp.1380-1402.
Stewart, D.L. (2021) ‘Performing goodness in qualitative research methods’, International Journal of Qualitative Studies in Education, 35(1), pp.58-70. Web.
Nyumba, T., Wilson, K., Derrick, C.J. and Mukherjee, N. (2018) ‘The use of focus group discussion methodology: Insights from two decades of application in conservation’, Methods in Ecology and evolution, 9(1), pp.20-32. Web.
Osborne, N. and Grant-Smith, D. (2021) ‘In-Depth Interviewing’, Methods in Urban Analysis (pp. 105-125). Springer. Web.
Rose, J. and Johnson, C.W. (2020) ‘Contextualizing reliability and validity in qualitative research: toward more rigorous and trustworthy qualitative social science in leisure research’, Journal of Leisure Research, 51(4), pp.432-451. Web.
Saarijärvi, M. and Bratt, E.L. (2021) When face-to-face interviews are not possible: tips and tricks for video, telephone, online chat, and email interviews in qualitative research. Web.
Subekti, A.S. (2021) ‘Covid-19-triggered online learning implementation: Pre-service English teachers’ beliefs’, Metathesis: Journal of English Language, Literature, and Teaching, 4(3), pp.232-248. Web.
Thompson, S. and Schick-Makaroff, K. (2021) ‘Qualitative research in clinical epidemiology’, Clinical Epidemiology (pp. 369-388). Humana, New York. Web.
Trang, N.M. (2022) ‘Using YouTube Videos to Enhance Learner Autonomy in Writing: A Qualitative Research Design’, Theory and Practice in Language Studies, 12(1), pp.36-45. Web.
Zwan, M.D et al. (2021) ‘Dutch Brain Research Registry for study participant recruitment: design and first results’, Alzheimer’s & Dementia: Translational Research & Clinical Interventions, 7(1), p.e12132. Web.